Christine Task - Knexus Research Corporation
Data, Privacy---and the Interactions Between Them
Nov 09, 2022
Download:

Abstract
Data deidentification aims to provide data owners with edible cake: to allow them to freely use, share, store and publicly release sensitive record data without risking the privacy of any of the individuals in the data set. And, surprisingly, given some constraints, that's not impossible to do. However, the behavior of a deidentification algorithm depends on the distribution of the data itself.
Privacy research often treats data as a black box---omitting formal data-dependent utility analysis, evaluating over simple homogeneous test data, and using simple aggregate performance metrics. As a result, there's less work formally exploring detailed algorithm interactions with realistic data contexts. This can result in tangible equity and bias harms when these technologies are deployed; this is true even of deidentification techniques such as cell-suppression which have been in widespread use for decades. At worst, diverse subpopulations can be unintentionally erased from the deidentified data.
Successful engineering requires understanding both the properties of the machine and how it responds to its running environment. In this talk I'll provide a basic outline of distribution properties such as feature correlations, diverse subpopulations, deterministic edit constraints, and feature space qualities (cardinality, ordinality), that may impact algorithm behavior in real world contexts. I'll then use new (publicly available) tools from the National Institute of Standards and Technology to show unprecedentedly detailed performance analysis for a spectrum of recent and historic deidentification techniques on diverse community benchmark data. We'll combine the two and consider a few basic rules that help explain the behavior of different techniques in terms of data distribution properties. But we're very far from explaining everything—I'll describe some potential next steps on the path to well-engineered data privacy technology that I hope future research will explore. A path I hope some CERIAS members might join us on later this year.
This talk will be accessible to anyone who's interested—no background in statistics, data, or recognition of any of the above jargon is required.
About the Speaker
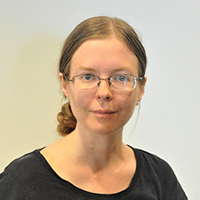
Ways to Watch
