Balamurugan Anandan - Purdue University
Students: Spring 2025, unless noted otherwise, sessions will be virtual on Zoom.
Secure Multiparty Computation and Differential Privacy
Nov 04, 2015
Download:

Abstract
Secure multiparty computation (MPC) and differential privacy are two notions of privacy that deal respectively with how and what functions can be privately computed. In this talk, I will first give an overview of MPC and differential privacy. Then, I will show how to build a two party differentially private secure protocol in the presence of semi-honest and malicious adversaries.Computing a differentially private function using secure function evaluation prevents private information leakage both in the process, and from information present in the function output. However, the very secrecy provided by secure function evaluation poses new challenges if any of the parties are malicious. We then relax the utility requirement of computational differential privacy to reduce computational cost, still giving security with rational adversaries. Finally, we provide a modified two-party computational differential privacy definition and show correctness and security guarantees in the rational setting.
About the Speaker
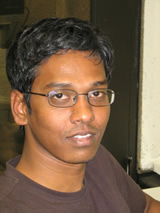
Ways to Watch
